The Role of AI in Data Science: Opportunities and Challenges
artificial intelligence, Data science, Navigation, UncategorizedIntroduction
Artificial intelligence (AI) is rapidly transforming the landscape of data science, ushering in an era of unprecedented opportunities while simultaneously presenting complex challenges. The synergy between these two fields is undeniable, with AI providing the tools and techniques to unlock the full potential of data, and data serving as the fuel that powers AI algorithms. This symbiotic relationship is revolutionizing how we collect, process, analyze, and interpret information, leading to breakthroughs across various industries. This article explores the multifaceted role of AI in data science, examining the exciting opportunities it presents and the significant challenges that must be addressed to ensure its responsible and effective implementation.
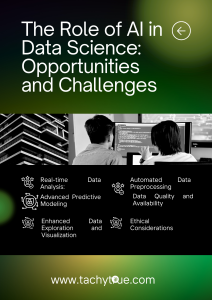
Opportunities: Unleashing the Power of Data
AI is empowering data science in several key areas, creating opportunities that were previously unimaginable:
Automated Data Preprocessing: Data preprocessing, a crucial but often tedious step in data science, involves cleaning, transforming, and preparing data for analysis. AI algorithms can automate many of these tasks, significantly reducing the time and effort required. For instance, AI-powered tools can identify and handle missing values, outliers, and inconsistencies in data, ensuring data quality and consistency. This automation frees up data scientists to focus on more strategic and creative aspects of their work.
Enhanced Data Exploration and Visualization: AI can facilitate more sophisticated data exploration and visualization. Machine learning algorithms can identify hidden patterns, correlations, and anomalies in data that might be missed by traditional methods. AI-powered visualization tools can then create interactive and insightful visualizations, enabling data scientists to better understand the data and communicate their findings effectively.
Advanced Predictive Modeling: Predictive modeling, a core component of data science, involves building models to predict future outcomes based on historical data. AI, particularly machine learning, has revolutionized predictive modeling by providing powerful algorithms that can learn complex patterns and relationships in data. This has led to more accurate and robust predictive models in various domains, such as finance, healthcare, and marketing.
Real-time Data Analysis: The ability to analyze data in real time is becoming increasingly important in many applications, such as fraud detection, stock trading, and traffic management. AI algorithms can process massive streams of data in real time, identifying patterns and anomalies as they occur. This enables organizations to make timely decisions and take immediate action based on the insights gained.
Improved Decision Making: Ultimately, the goal of data science is to improve decision making. AI is playing a crucial role in this by providing data-driven insights that can inform strategic and operational decisions. AI-powered decision support systems can analyze complex scenarios, evaluate different options, and recommend the most effective course of action.
Challenges: Navigating the Complexities of AI in Data Science
Despite the immense opportunities, the integration of AI into data science also presents significant challenges:
Data Bias and Fairness: AI algorithms are only as good as the data they are trained on. If the data contains biases, the resulting AI models will also be biased, leading to unfair or discriminatory outcomes. Addressing data bias and ensuring fairness in AI algorithms is a critical challenge that requires careful attention to data collection, preprocessing, and model development.
Explainability and Interpretability: Many AI algorithms, particularly deep learning models, are often considered “black boxes,” meaning that their decision-making processes are opaque and difficult to understand. This lack of explainability can be a barrier to trust and adoption, especially in critical applications where transparency is essential. Developing more explainable and interpretable AI models is an important area of research.
Data Security and Privacy: AI algorithms often require vast amounts of data, raising concerns about data security and privacy. Protecting sensitive data from unauthorized access and ensuring compliance with privacy regulations are crucial challenges. Techniques like differential privacy and federated learning are being developed to address these concerns.
Computational Resources: Training and deploying complex AI models can require significant computational resources, including powerful hardware and specialized software. The development of more efficient AI algorithms and the availability of cloud-based computing platforms are helping to mitigate this challenge.
Skills Gap: The rapid advancement of AI and data science has created a skills gap, with a shortage of professionals who have the necessary expertise to develop and implement AI-powered solutions. Bridging this skills gap through education and training is essential for realizing the full potential of AI in data science.
Ethical Considerations: The use of AI in data science raises various ethical considerations, such as the potential for job displacement, the misuse of AI for malicious purposes, and the impact of AI on human autonomy. Addressing these ethical considerations and developing guidelines for responsible AI development and deployment are crucial.
Data Quality and Availability: The success of AI in data science depends heavily on the availability of high-quality data. In many cases, data may be incomplete, inconsistent, or unavailable, making it difficult to train effective AI models. Improving data quality and accessibility is a crucial prerequisite for leveraging AI in data science.
The Future of AI in Data Science:
Despite the challenges, the future of AI in data science is bright. As AI technologies continue to advance and data becomes more readily available, we can expect to see even more transformative applications of AI in data science. Addressing the challenges outlined above will be crucial for ensuring that AI is used responsibly and ethically, maximizing its benefits while minimizing its risks. The convergence of AI and data science is not just a technological trend; it is a fundamental shift in how we understand and interact with the world around us. By embracing the opportunities and addressing the challenges, we can unlock the full potential of this powerful combination and create a future where data-driven insights drive innovation and progress across all aspects of society.